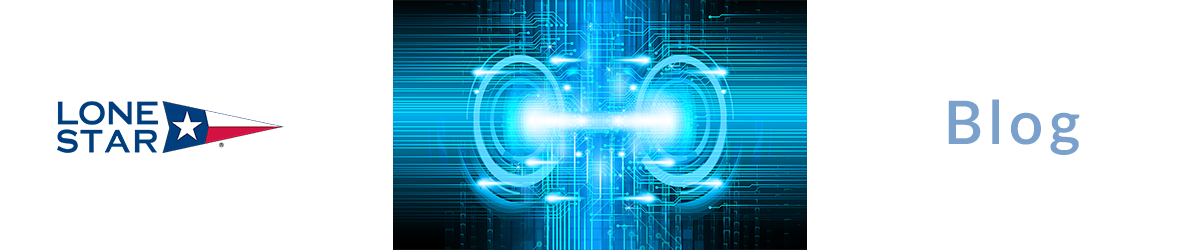
Opportunities and Problems with Digital Twins | Part Three: Barriers and Business Models
So far, this series has discussed the problems of confusion about different kinds of digital twins and other ways they generate value. This final installment considers a separate issue. Like many technology offerings, there are interesting relationships between business models to capture the value and the barriers preventing adoption.
Digital Twins Barriers and Business Models
The list of issues to consider is too long for this short article, but there are four questions that seem to address the majority of barriers and risks. Of course, there are others. Many barriers are common to Analytics and Data Science in general, not restricted to Digital Twins.
Who Benefits?
Perhaps the most common barrier to Digital Twin adoption is a mismatch between who pays and who benefits.
An OEM (Original Equipment Manufacturer) can build a twin into a product, but who benefits? Is it the retailer who sells the product? The end-user? It’s crucial to ask “who benefits” and determine whether there is a connection between the “who benefits” and the maker of the twins.
Large data flows can tax the organization’s IT infrastructure in a corporation. The Chief Information Officer generally gets the bill for this. But the benefits probably accrue to the operating units. This problem is particularly acute as companies move to the cloud. Cloud costs are based on complex usage models. When digital twins change the kinds and magnitude of usage, someone will get a bill they didn’t expect. The odds are good that the billpayer is not the one benefiting.
For example, the sales team focuses on the CIO in a large Systems Integration firm selling IT services. Salespeople focus on “who pays,” but the “who benefits” is in a part of the company they rarely see. So, the selling team is likely to be ineffective.
This “who benefits” question helps illuminate systematic weaknesses in the buyers, sellers, and users of Digital Twins. This is not a new idea; it has slowed the adoption of other technologies. But the case of Digital Twins may be a particularly bad example of this problem.
Starting small, without the need to pull data across multiple boundaries, is often the best approach.
Where is the data?
We’ve seen how benefits and payment can reside in different stovepipes. The same thing can happen with data. Sales data, failure data, configuration data – all these are likely to reside in different systems with different organizational owners. Finding it can be a challenge, and when finally found, we will discover all kinds of incompatibilities. The stovepipe owners will debate who should change, who controls the changes, who gets access, who pays for changes, who pays for access, who pays for cloud costs, and so on.
Most large organizations have some inkling of the data stovepipe challenge. They’ve struggled with it before they thought about Digital Twins. But some kinds of Digital Twins bring new kinds of “where is the data” problems.
Your suppliers illustrate the connection between “who pays” and “where is the data.” Most firms offering a product (whether physical or virtual) depend on lower-tier suppliers to provide components, software, and services. For instance, a Ford F-150 has physical parts from more than a dozen vendors. The truck’s software comes from many suppliers. It depends on services like SiriusXM and mobile data connections. An F-150 Digital Twin probably needs data from (or about) these suppliers. They aren’t going to give it up willingly in many cases.
For MAIM (Mainstream AI Methods), we typically need large quantities of labeled training data. Some retail and consumer-facing firms can generate this. When we say a company was “born digital,” we often mean coherent data collection, labeling and use were foundational considerations.
But most industrial and infrastructure firms were NOT born digital.
When you hire your fancy new data scientist, the expensive new pro might ask your most valuable people to label data. Since there is a LOT of data to label, and your valuable folks have other things to do, you might be asking, “where is the data” for a long time.
Pure data-driven Digital Twin technology often dies because of this issue. It’s a barrier to some kinds of “mimic twins” and nearly all twins based on MAIM. One reason why rule-based twins (e.g., UML mimic twins) can be a better choice.
This issue is also why some sophisticated firms adopt hybrid twins, using AI to “learn” only what they don’t know already. This approach is one of Lone Star’s preferred approaches with our Evolved AI® and Deep Evolved AI® (and how our MaxUp® CBM twin of your F-150 overcomes these barriers).
When is the data?
So, it should be clear that “where is the data” is closely related to asking “when” we can use it. This is one form of the “when is the data” question. Unfortunately, it’s not the only timing-related problem.
- When was it created? Timestamps are important for many twin applications. Timing is also important when we need to synchronize data from multiple sources (stovepipes).
- Does it still matter? Airline passenger behavior information before March 2020 was extremely valuable in creating twins of passenger personas. But as the Covid-19 epidemic emerged, the historical data was useless. Things change over time, people change over time, and your twins need to reflect these changes. Twins based on data only are weak in this area. Major events cause twins to fail if this issue is ignored.
- When was the recorded time added? If a timestamp is “when received,” it was created earlier. How much earlier? Milliseconds? Weeks?
- When do you need a twin’s response? If you require notification of a pending failure three weeks before that event, you probably need data feed in near real-time. If you need six months of lead time to order parts from your supply chain, a day or two delays in the data might not matter.
Generally, the more data-hungry your technology is, the more we should expect timing issues. This is a consideration for both the Digital Twin architecture and the architecture of the overall solution (edge, cloud).
Starting with use cases, which can succeed despite your timing issues, is usually best. Other use cases can come later.
How soon can we start?
The time value of money is a real consideration for most organizations. This is a significant barrier for MAIM-based twins.
Starting soon, with a bias for action drives returns and organizational learning. Benefits like this help weaken stovepipes and other barriers.
Tags In
Recent Posts
- Lone Star Analysis Awarded Digital Twin Patent for Operations and Maintenance Applications
- The Steps to Laying Out an Embedded System Quantum Roadmap Featured in Military Embedded Systems
- Steve Roemerman Recognized as Aerospace AI Leader in Inaugural AI 75 List
- Lone Star Analysis Ltd. Sponsoring RAFA Rides 2024
- The Power of Predictive Modeling in Complex Problem Solving featured in COTS Journal