Second Unsolved Problem in Data Science and Analytics
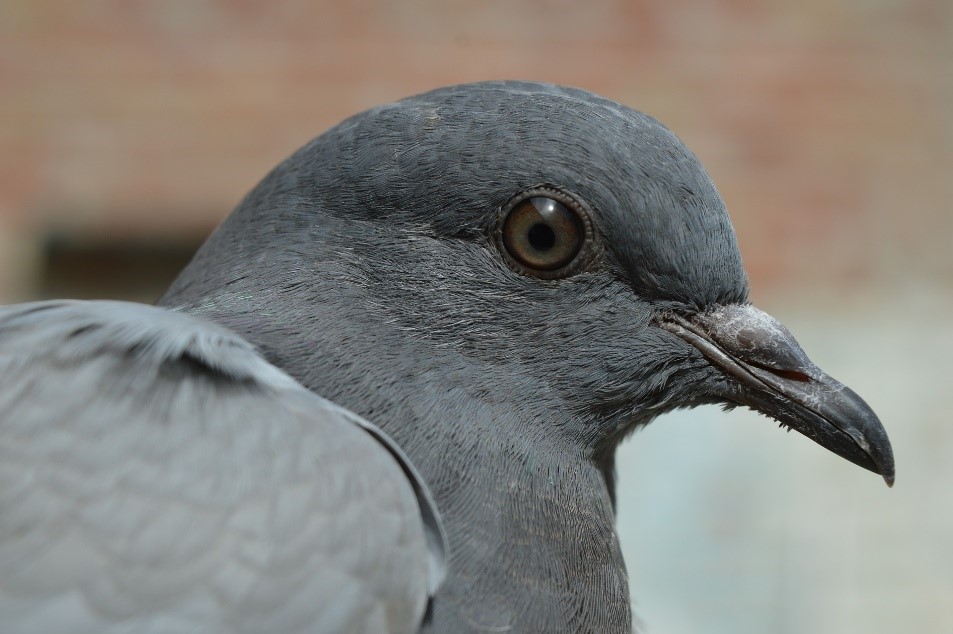
Our second unsolved problem; correctly dealing with uncertainty.
In a way the problem is us humans. We are bad at dealing with uncertainty. Our friend on the left is a pigeon whose brain is wired for it. Some wonderful work by Walter Herbranson at Whitman College shows when it comes to reasoning in the face of uncertainty pigeons are better than most humans, including most math professors.
Our brains are just not wired for it. Even when we SEE the data. Lone Star has done research on how humans express uncertainty, how we understand it, and how we make decisions in the face of it. Our work showed people are only good at this under very narrow conditions.
For example, they can only reliably answer Little Questions™. And, while our work (and work done by Doug Hubbard) shows that training can improve most people’s ability to estimate spans of uncertainty, that ONLY applies to these narrow, Little Questions. In fact, our research shows university education in probability and statistics doesn’t help people improve much, and may make some of us dumber.
No matter how gifted you are, you probably aren’t very good at this.
Humans who are compared to pigeons in Herbranson’ s research are much better at probabilistic reasoning.
Don’t feel bad. The problem Herbranson uses for pigeons stumped Paul Erdos. Erdos was the most prolific mathematician in history. He published about 1500 papers before he died in 1996. Some of his important work was in probabilistic methods. But, according to his biographer, he refused to believe the correct solution until shown the results of a Monte Carlo simulation.
If Erdos had a hard time being intuitive with the mathematics of uncertainty the rest of us don’t have much of a chance. Yet we must make decisions which incorporate uncertainty.
So, an unsolved problem is how to deal correctly with uncertainty when we do analytics and data science. This is really a collection of problems. Some are procedural, some related to visualizations, some are rooted in how we teach our students.
A great deal of this problem seems to relate to information theory and signal processing.
Those two disciplines have struggled for about 80 years with the problems of randomness and noise. We can learn a lot from them.
But we see data science practitioners “clean” their data without good rationale about what is “dirt” and what is “noise”, so it seems data science can still learn from signal processing and information theory. This problem is connected to our first problem; detecting and classifying types of data dirt.
In a global benchmarking project, we found this area to be one of the most troublesome. Spans of uncertainty are collected, ingested for analytics, computed, reported and used. At each step, benchmarking shows there are real challenges.
Dealing with uncertainty is the second unsolved problem.
About Lone Star Analysis
Lone Star Analysis enables customers to make insightful decisions faster than their competitors. We are a predictive guide bridging the gap between data and action. Prescient insights support confident decisions for customers in Oil & Gas, Transportation & Logistics, Industrial Products & Services, Aerospace & Defense, and the Public Sector.
Lone Star delivers fast time to value supporting customers planning and on-going management needs. Utilizing our TruNavigator® software platform, Lone Star brings proven modeling tools and analysis that improve customers top line, by winning more business, and improve the bottom line, by quickly enabling operational efficiency, cost reduction, and performance improvement. Our trusted AnalyticsOSSM software solutions support our customers real-time predictive analytics needs when continuous operational performance optimization, cost minimization, safety improvement, and risk reduction are important.
Headquartered in Dallas, Texas, Lone Star is found on the web at http://www.Lone-Star.com.